Artificial Intelligence (AI) has the potential to support Environmental, Social and Governance (ESG) initiatives in a number of ways.
Artificial intelligence (AI) has the potential to revolutionize ESG investing by providing new insights into environmental, social, and governance factors. Here are a few ways in which AI is being used in ESG:

Environmental impact:
Artificial intelligence can be used to monitor and analyze large amounts of data on the environment, such as satellite imagery and sensor data, to identify patterns and trends related to climate change, biodiversity, and other environmental issues. This can help to support decision-making and planning related to sustainable development, conservation, and climate change adaptation.
Social impact:
We are already seeing ways is improving access to services and support for vulnerable communities, such as through the development of chatbots or virtual assistants that can provide information and assistance related to health care, education, and other services. AI can also be used to improve the efficiency and effectiveness of social services, such as through the use of predictive analytics to identify individuals and communities at risk of poverty or social exclusion.
Governance impact:
AI can be used to promote transparency and accountability in government and other organizations, such as through the use of natural language processing to analyze and summarize large amounts of text data, such as news articles and social media posts. It can also be used to support decision-making in government and other organizations, such as through the use of machine learning algorithms to analyze data on public opinion, social trends, and other factors.
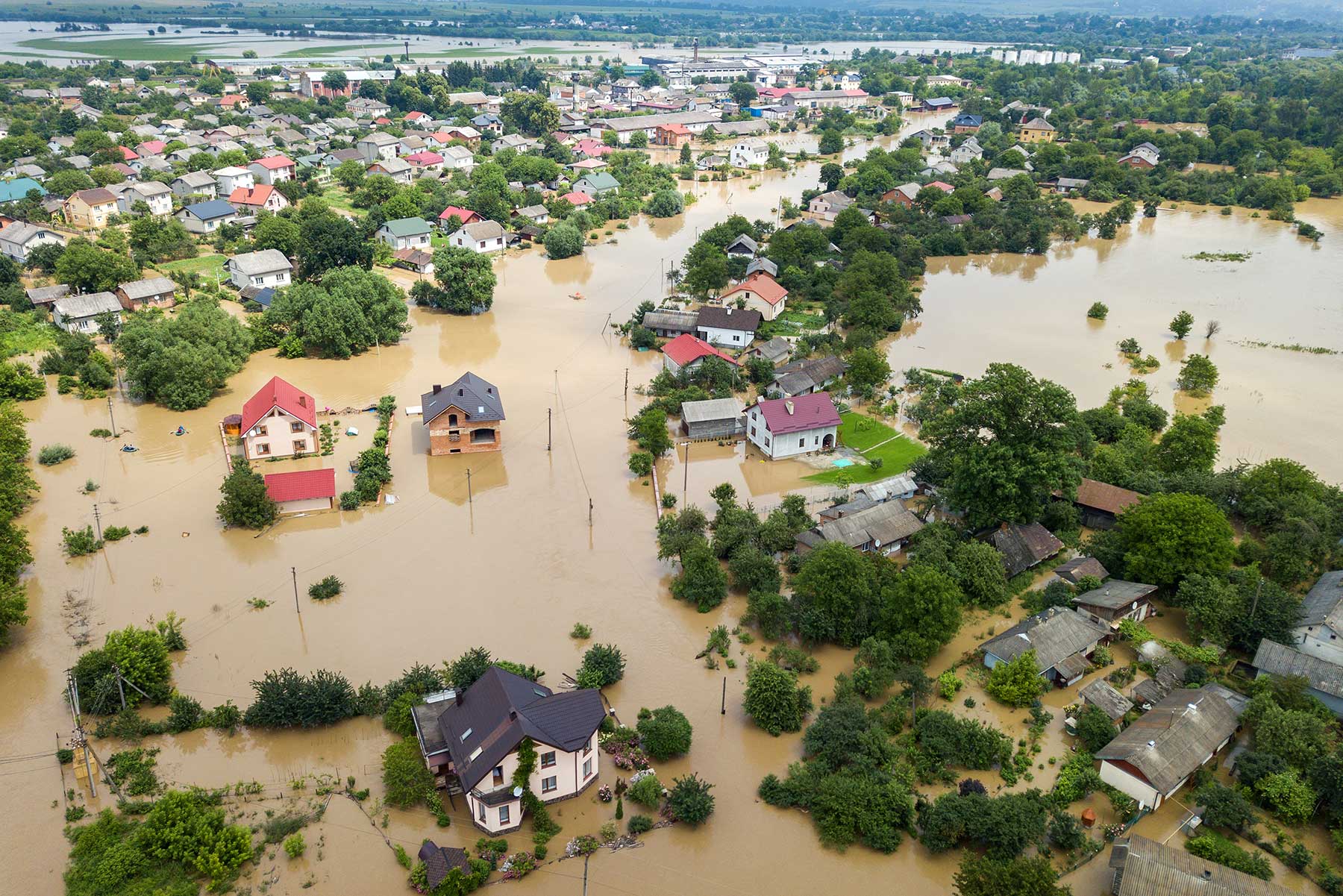
Data analysis and Climate risk modeling
AI can also be used to model the potential impact of climate change on a company's operations and financial performance. By analyzing climate data, satellite imagery, and other sources, AI algorithms can help investors understand the physical risks of climate change, such as sea-level rise and extreme weather events, and the transition risks, such as policy changes and shifts in consumer behavior.
Machine learning algorithms can analyze satellite imagery to monitor deforestation rates or carbon emissions from factories allowing stakeholders to gain deeper insights from climate risk models. For example, the AI-powered platform, Four Twenty Seven, uses machine learning algorithms to analyze climate data and to provide risk assessments for physical climate risks such as sea level rise, extreme heat, and hurricanes.
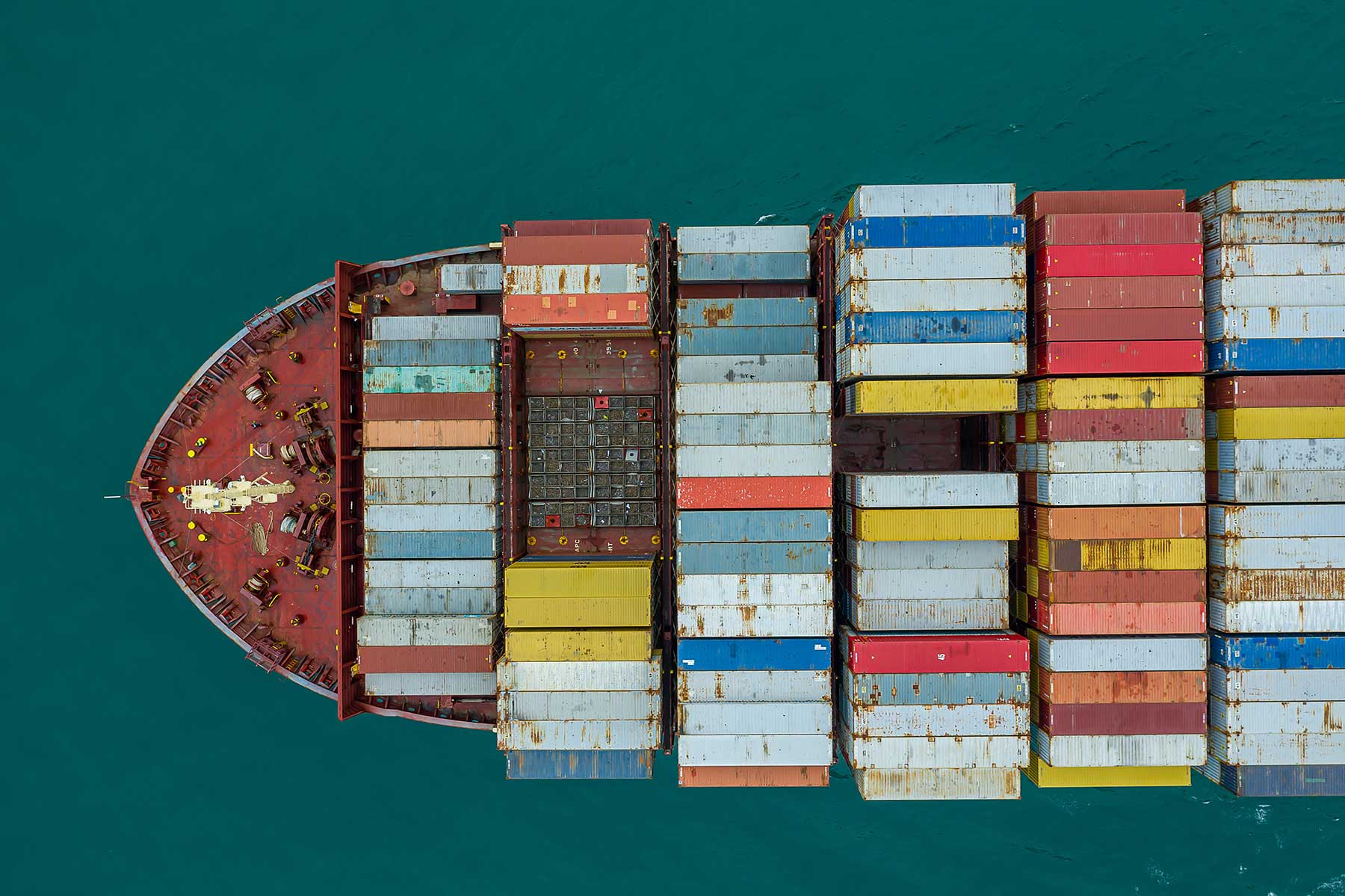
Supply Chain Management:
AI algorithms can be used to track and analyze the environmental and social impacts of supply chains, such as identifying potential risks related to human rights violations, child labor, or deforestation and helping to identify areas for improvement. Provenance, uses blockchain technology to track and verify the social and environmental impact of products throughout the supply chain.
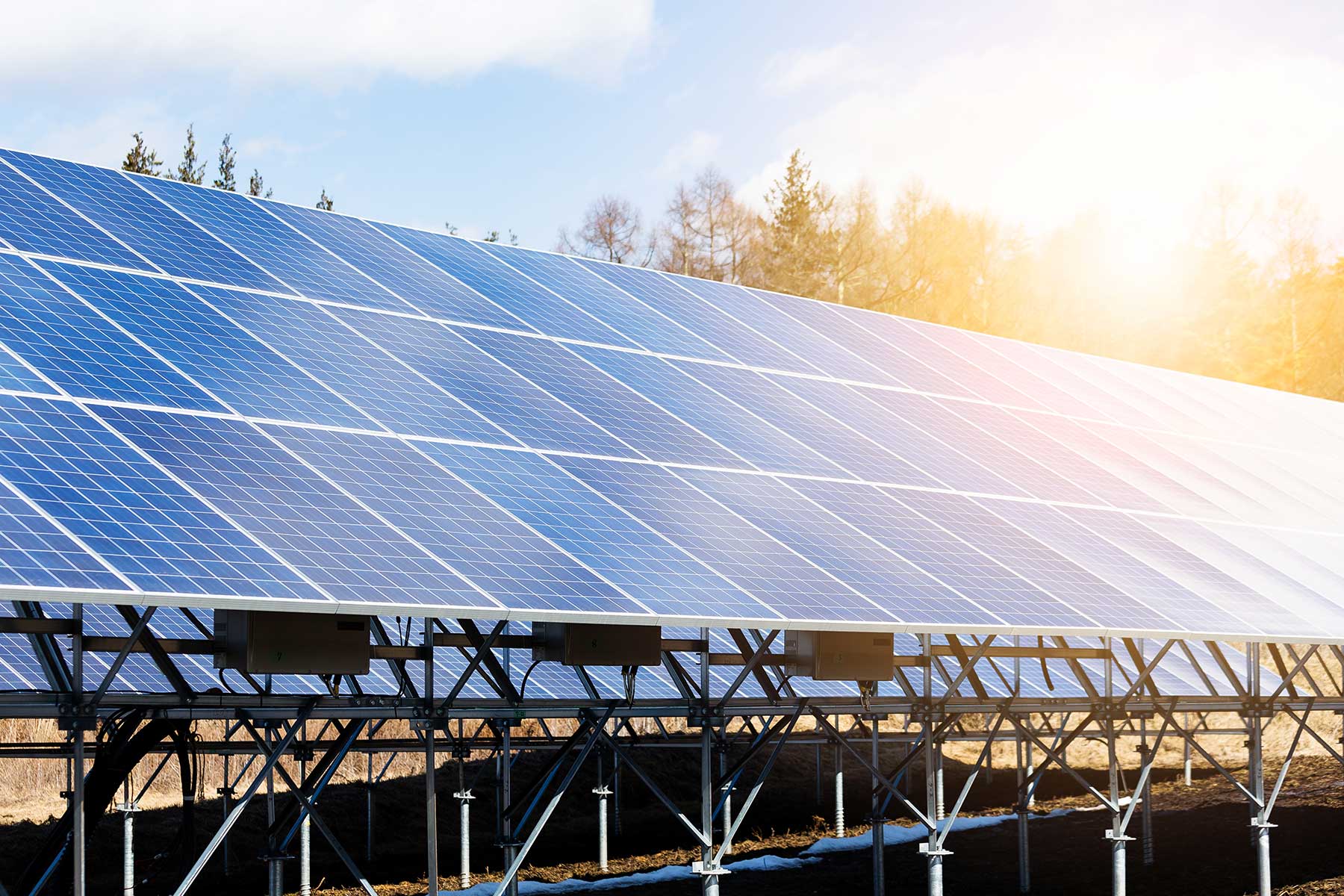
Renewable Energy:
AI is being used to improve the efficiency and effectiveness of renewable energy systems worldwide. Wind farms are a key source of renewable energy, but they can be inefficient and costly to operate if they're not optimized properly. To address this challenge, companies like Google are using AI to improve the efficiency of wind farms.
Google's subsidiary, DeepMind, developed an AI system that uses machine learning algorithms to predict wind output from wind farms up to 36 hours in advance. The system uses weather forecasts, historical data, and real-time data from sensors on the wind turbines to make predictions.
By predicting wind output more accurately, the AI system can help wind farm operators optimize their operations and increase their energy output. This can help reduce the cost of generating renewable energy and make wind energy more competitive with traditional energy sources. In 2019, Google announced that it had used the AI system to increase the value of its wind energy by 20 percent, and that it planned to share the technology with other wind farm operators.
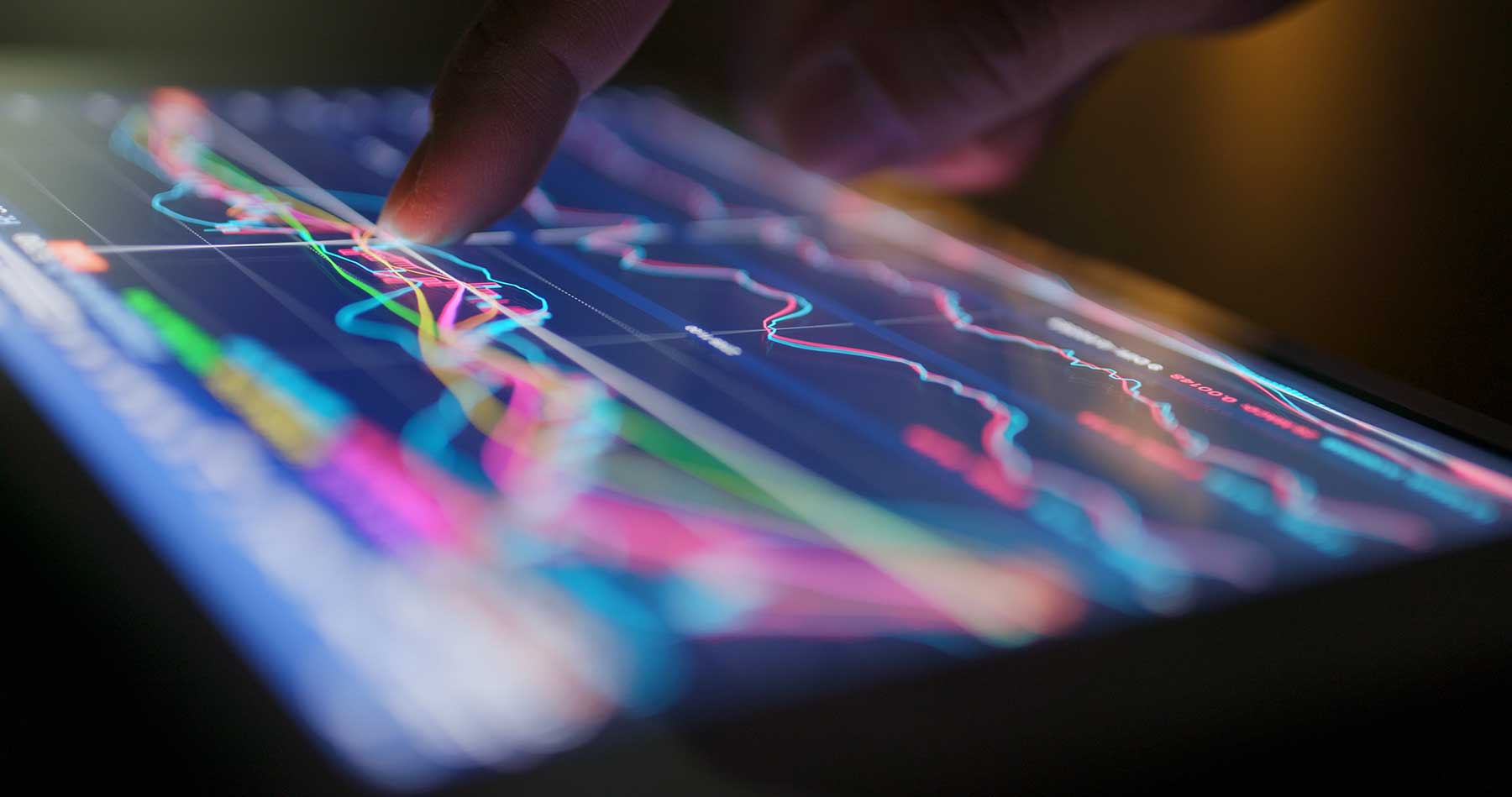
ESG ratings:
AI can by applied by ESG rating agencies to provide more accurate and reliable ESG ratings. Machine learning algorithms can analyze large amounts of data to identify patterns and correlations between ESG factors and financial performance, which can help rating agencies to better assess a company's ESG performance. One example of this is Arabesque S-Ray, which uses natural language processing and machine learning algorithms to analyze ESG data from a range of sources and to provide investors with real-time ESG scores for companies.
ESG ratings, scoring and reporting:
The FUTR PROOF AI (Genesis) currently in development uses a combination of quantitative and qualitative data, AI algorithms to provide more accurate and consistent ESG ratings than traditional methods
These are just a few examples of how AI is being used in ESG. As AI technology continues to advance, we can expect to see even more innovative applications of AI in the ESG space.